forked from glen/dyna3
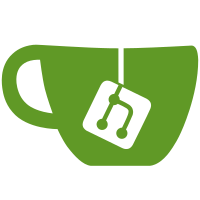
The completed gram matrix from this commit matches the one from commit
e7dde58
to six decimal places.
100 lines
No EOL
2.6 KiB
Julia
100 lines
No EOL
2.6 KiB
Julia
module Engine
|
|
|
|
using LinearAlgebra
|
|
using SparseArrays
|
|
|
|
export Q, DescentHistory, realize_gram
|
|
|
|
# the Lorentz form
|
|
Q = diagm([1, 1, 1, 1, -1])
|
|
|
|
# the difference between the matrices `target` and `attempt`, projected onto the
|
|
# subspace of matrices whose entries vanish at each empty index of `target`
|
|
function proj_diff(target::SparseMatrixCSC{T, <:Any}, attempt::Matrix{T}) where T
|
|
J, K, values = findnz(target)
|
|
result = zeros(size(target)...)
|
|
for (j, k, val) in zip(J, K, values)
|
|
result[j, k] = val - attempt[j, k]
|
|
end
|
|
result
|
|
end
|
|
|
|
# a type for keeping track of gradient descent history
|
|
struct DescentHistory{T}
|
|
scaled_loss::Array{T}
|
|
stepsize::Array{T}
|
|
backoff_steps::Array{Int64}
|
|
|
|
function DescentHistory{T}(
|
|
scaled_loss = Array{T}(undef, 0),
|
|
stepsize = Array{T}(undef, 0),
|
|
backoff_steps = Int64[]
|
|
) where T
|
|
new(scaled_loss, stepsize, backoff_steps)
|
|
end
|
|
end
|
|
|
|
# seek a matrix `L` for which `L'QL` matches the sparse matrix `gram` at every
|
|
# explicit entry of `gram`. use gradient descent starting from `guess`
|
|
function realize_gram(
|
|
gram::SparseMatrixCSC{T, <:Any},
|
|
guess::Matrix{T};
|
|
scaled_tol = 1e-30,
|
|
target_improvement = 0.5,
|
|
init_stepsize = 1.0,
|
|
backoff = 0.9,
|
|
max_descent_steps = 600,
|
|
max_backoff_steps = 110
|
|
) where T <: Number
|
|
# start history
|
|
history = DescentHistory{T}()
|
|
|
|
# scale tolerance
|
|
scale_adjustment = sqrt(T(nnz(gram)))
|
|
tol = scale_adjustment * scaled_tol
|
|
|
|
# initialize variables
|
|
stepsize = init_stepsize
|
|
L = copy(guess)
|
|
|
|
# do gradient descent
|
|
Δ_proj = proj_diff(gram, L'*Q*L)
|
|
loss = norm(Δ_proj)
|
|
for step in 1:max_descent_steps
|
|
# stop if the loss is tolerably low
|
|
if loss < tol
|
|
break
|
|
end
|
|
|
|
# find negative gradient of loss function
|
|
neg_grad = 4*Q*L*Δ_proj
|
|
slope = norm(neg_grad)
|
|
|
|
# store current position and loss
|
|
L_last = L
|
|
loss_last = loss
|
|
push!(history.scaled_loss, loss / scale_adjustment)
|
|
|
|
# find a good step size using backtracking line search
|
|
push!(history.stepsize, 0)
|
|
push!(history.backoff_steps, max_backoff_steps)
|
|
for backoff_steps in 0:max_backoff_steps
|
|
history.stepsize[end] = stepsize
|
|
L = L_last + stepsize * neg_grad
|
|
Δ_proj = proj_diff(gram, L'*Q*L)
|
|
loss = norm(Δ_proj)
|
|
improvement = loss_last - loss
|
|
if improvement >= target_improvement * stepsize * slope
|
|
history.backoff_steps[end] = backoff_steps
|
|
break
|
|
end
|
|
stepsize *= backoff
|
|
end
|
|
end
|
|
|
|
# return the factorization and its history
|
|
push!(history.scaled_loss, loss / scale_adjustment)
|
|
L, history
|
|
end
|
|
|
|
end |